Artificial Intelligence (AI) is a vast and multifaceted field, encompassing various domains that contribute to the development of intelligent systems. These domains highlight the versatility and breadth of AI technologies, showcasing their applications across different sectors. In this blog post, we will explore the primary domains of AI, discussing their unique features, methodologies, and applications.
1. Perception
Perception in AI involves interpreting data from the world using sensory inputs. This domain mimics human senses like sight, sound, and touch, enabling machines to understand and interact with their environment.
Key Areas
- Computer Vision: This area allows machines to interpret and process visual information. Techniques like image classification, object detection, and facial recognition are central to computer vision, enabling applications in security and healthcare fields.
- Speech Recognition: Converts spoken language into text, facilitating voice-activated systems and virtual assistants. This technology is crucial for enabling hands-free operation and accessibility features in devices.
- Audio Processing analyzes sound patterns to recognize specific acoustics, which is essential in applications like music recognition and environmental sound analysis. This area helps create immersive audio experiences and enhances hearing aids.
Applications
Perception is utilized in autonomous vehicles to detect obstacles, in security systems for surveillance, and in assistive technologies to aid the visually impaired. These applications demonstrate the potential of perception technologies to enhance safety and accessibility in various contexts.
2. Reasoning and Problem Solving
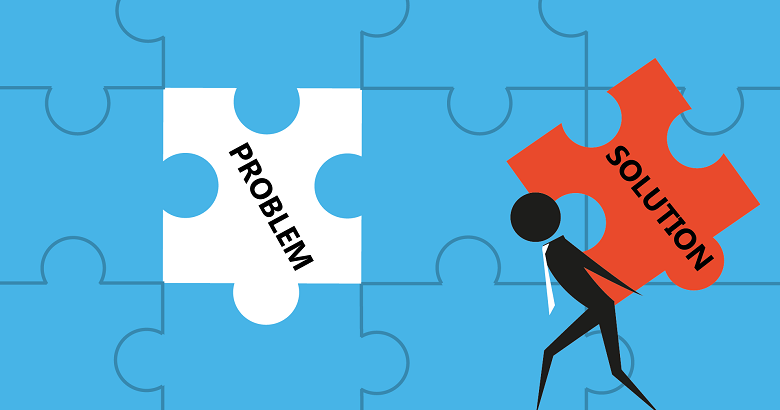
This domain focuses on enabling machines to think logically and solve complex problems. It involves developing algorithms that can simulate human reasoning, allowing AI systems to make informed decisions.
Key Areas
- Expert Systems: These systems are designed to mimic human decision-making by using a set of rules and facts to solve specific problems. They are often used in medical diagnosis and financial forecasting, providing expert-level insights and recommendations.
- Automated Planning: Involves creating sequences of actions to achieve specific goals. This area is crucial in robotics and logistics, where efficient planning can significantly enhance operational efficiency.
- Optimization: Techniques that find the best solution among many possible options. Optimization is widely used in resource allocation, scheduling, and supply chain management, helping organizations maximize efficiency and reduce costs.
Applications
Reasoning and problem-solving are integral to strategic gameplay, where AI systems like those used in chess and Go demonstrate advanced decision-making capabilities. In business, these domains are applied to optimize operations and enhance decision-making processes.
3. Learning
Learning in AI refers to the ability of systems to improve their performance based on experience. This domain is primarily driven by machine learning techniques, which enable AI systems to adapt and evolve.
Key Areas
- Supervised Learning: Models learn to make predictions or classifications from labeled data. This technique is widely used in applications like spam detection, image recognition, and predictive analytics.
- Unsupervised Learning: This technique identifies patterns and structures in unlabeled data, which is useful for clustering and anomaly detection. It is also employed in customer segmentation and market analysis.
- Reinforcement Learning: Involves training models to make decisions by rewarding desired actions. This technique is prominent in autonomous vehicles and gaming, where AI systems learn optimal strategies through trial and error.
Applications
Learning technologies are used to create personalized recommendations, such as those used by streaming services and online retailers. They are also crucial in fraud detection, where AI systems identify unusual patterns in financial transactions.
4. Natural Language Processing (NLP)
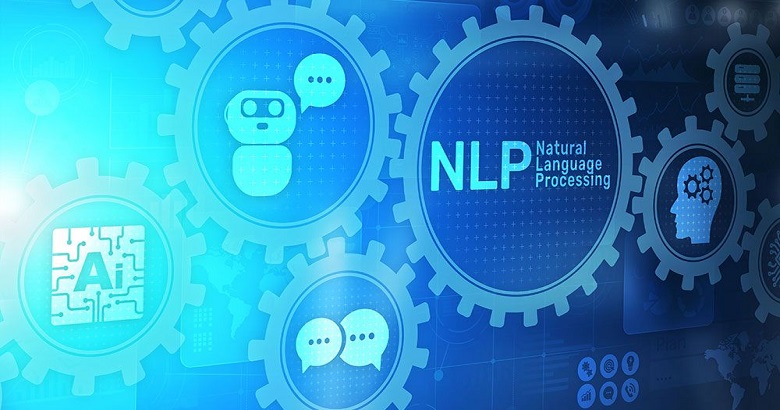
NLP enables machines to understand, interpret, and generate human language. This domain bridges the gap between human communication and computer understanding, making machine interactions more intuitive.
Key Areas
- Text Analysis involves extracting meaningful information from text, such as sentiment analysis and keyword extraction. It is used in content moderation and social media monitoring.
- Machine Translation: Automatically translates text from one language to another, playing a vital role in breaking down language barriers and fostering global communication.
- Chatbots and Conversational Agents: Facilitate human-like interactions with machines, providing customer support and information retrieval services.
Applications
NLP is crucial for automating customer service, enabling real-time translation services, and enhancing content discovery through intelligent search engines. These applications demonstrate the impact of NLP on improving communication and accessibility.
5. Robotics
Robotics is the domain where AI intersects with mechanical engineering to create machines that can perform tasks autonomously. This area focuses on designing robots that can navigate and interact with their environments.
Key Areas
- Robotic Process Automation (RPA): Automates repetitive tasks in business processes, enhancing efficiency and reducing human error.
- Autonomous Robots: These robots navigate and interact with their environment without human intervention. They are used in various industries, from manufacturing to agriculture.
- Human-Robot Interaction: This area focuses on improving communication and collaboration between humans and robots, ensuring safety and effectiveness in shared spaces.
Applications
Robotics is used in manufacturing to assemble products, in healthcare to perform surgeries, and in service industries to perform tasks like cleaning and delivery. These applications highlight the versatility of robotics in automating tasks and enhancing productivity.
6. Social Intelligence
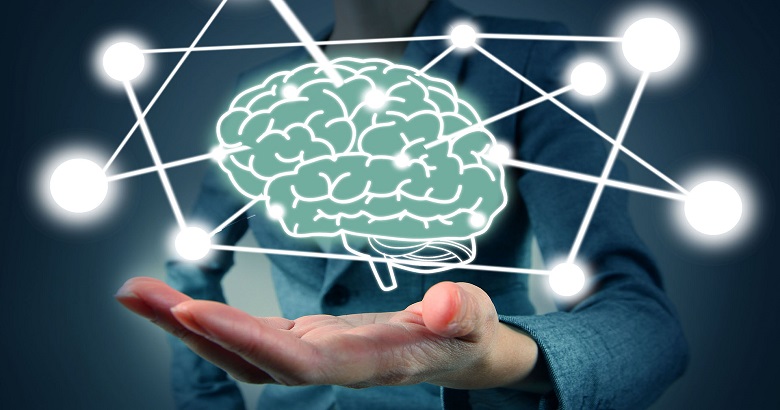
Social Intelligence involves creating systems that can understand and interact with humans in socially appropriate ways. This domain focuses on emotional recognition and social behavior modeling.
Key Areas
- Emotion Recognition: This technology identifies human emotions through facial expressions, voice, and body language. It is used in customer service to gauge customer satisfaction and tailor responses accordingly.
- Social Simulation: This technique models human social behaviors to predict outcomes in group interactions, and it is useful in sociology and urban planning.
Applications
Social intelligence is used in virtual reality environments to create realistic avatars that respond to user emotions. It is also applied in educational tools that tailor content to students’ emotional states, enhancing learning experiences.
7. General AI
General AI, also known as Artificial General Intelligence (AGI), aims to create machines that can understand, learn, and apply intelligence across various tasks, similar to human cognitive abilities.
Challenges
- Versatility: Unlike narrow AI, which excels in specific tasks, AGI must perform well across diverse domains. This requires a more holistic approach to intelligence.
- Adaptability: AGI systems must learn and adapt to new situations without extensive reprogramming, posing significant technical challenges.
Applications
While AGI remains largely theoretical, it represents the ultimate goal of many AI researchers. It promises advancements in areas like creativity, problem-solving, and ethical decision-making. The development of AGI could revolutionize industries by providing systems that can handle complex, multifaceted tasks.
8. Cognitive Computing
Cognitive Computing attempts to simulate human thought processes in a computerized model, using self-learning algorithms to mimic the human brain.
Key Areas
- Cognitive Analytics: Combines AI and data analytics to derive insights from complex data sets. This area is crucial for gaining a deeper understanding of consumer behavior and market trends.
- Machine Reasoning: This field focuses on reasoning and decision-making processes, enabling AI systems to provide insights beyond mere data analysis.
Applications
Cognitive computing is used in healthcare to create personalized treatment plans. AI systems analyze patient data to recommend customized therapies. It is also used in finance to manage risk, helping organizations assess and mitigate potential threats.
Wrapping Up
The domains of AI are diverse and continuously evolving, each contributing uniquely to the development of intelligent systems. From perception and learning to social intelligence and general AI, these domains illustrate the vast potential of AI technologies. These domains will likely intersect as AI advances, leading to more sophisticated and capable systems that can transform industries and improve our daily lives. Understanding these domains provides valuable insights into how AI can be harnessed to solve complex problems and drive innovation across various sectors. Whether enhancing human capabilities or automating routine tasks, AI remains a powerful force shaping the future of technology and society.
Frequently Asked Questions
1. How does AI perception differ from human perception?
AI perception relies on algorithms to process and interpret sensory data, focusing on pattern recognition and data-driven insights. While AI excels in analyzing large volumes of data quickly and accurately, it lacks the subjective interpretation and emotional context that human perception provides. Humans draw on experiences, emotions, and intuition to perceive and understand the world, adding layers of complexity that AI systems are still striving to replicate.
2. What challenges exist in developing General AI?
General AI, or Artificial General Intelligence (AGI), aims to replicate the broad cognitive abilities of humans, enabling machines to perform any intellectual task a human can do. The primary challenges include creating systems that can learn and adapt across various tasks without specific programming. This requires advancements in machine learning algorithms, understanding human cognition, and developing ethical frameworks to ensure AGI systems act responsibly and safely in diverse scenarios.
3. How is social intelligence integrated into AI systems?
Social intelligence in AI involves understanding and responding to human emotions and social cues. This is achieved through emotion recognition technology, which analyzes facial expressions, voice tone, and body language. AI systems use this data to engage in more natural and empathetic interactions, making them effective in customer service, education, and therapy applications. The integration of social intelligence aims to create AI that can build rapport and understand the nuances of human communication.
4. What role does cognitive computing play in healthcare?
Cognitive computing in healthcare involves using AI to simulate human thought processes for data analysis and decision-making. It helps process vast amounts of medical data, including patient records, research papers, and clinical trial results, to provide personalized treatment recommendations. This enhances healthcare professionals’ ability to diagnose diseases accurately, predict patient outcomes, and develop tailored treatment plans, ultimately improving patient care and operational efficiency.
5. Why is natural language processing crucial for AI advancement?
Natural Language Processing (NLP) enables machines to understand, interpret, and generate human language. This capability is crucial for creating intuitive human-machine interactions, such as voice-activated assistants, chatbots, and real-time translation services. NLP expands AI’s accessibility, allowing it to be integrated into everyday applications, enhancing user experience, and providing valuable insights from unstructured data sources like social media and customer feedback.