Artificial Intelligence (AI) has rapidly evolved, integral to various industries and everyday life. Understanding the diverse types of AI is essential, as they vary in complexity, capability, and application. This blog post explores the primary categories of AI, their characteristics, and how they impact our world.
Narrow AI
Narrow AI, or Weak AI, is designed to perform a specific task. It is currently the most common type of AI behind many daily technologies. Despite its name, Narrow AI can be incredibly powerful within its domain.
Examples of Narrow AI
- Voice Assistants: Siri, Alexa, and Google Assistant use AI to understand and respond to voice commands. These systems rely on natural language processing (NLP) to interpret spoken language and provide relevant responses. They are continuously improving, learning from interactions to better serve users.
- Recommendation Systems: Platforms like Netflix and Amazon employ AI algorithms to suggest content and products based on user behavior. By analyzing viewing habits, purchase history, and preferences, these systems enhance user experience and increase engagement.
- Image Recognition: Technologies like Facebook’s tagging feature and security surveillance systems utilize AI to identify and categorize images. This involves complex algorithms detecting faces, objects, and even emotions in photos.
- Chatbots: Many websites use customer service bots to handle inquiries and provide support. These chatbots can answer frequently asked questions, guide users through processes, and even process transactions, enhancing customer service efficiency.
Narrow AI excels at its designated function but cannot perform tasks outside its specific domain. This type of AI is prevalent due to its effectiveness in handling repetitive and defined tasks.
General AI
General AI, or Strong AI, refers to systems that can understand, learn, and apply knowledge across various tasks, similar to humans. General AI remains largely theoretical, as no current AI systems possess this level of understanding or adaptability.
Characteristics of General AI
- Human-like Cognitive Abilities: General AI would be capable of reasoning, problem-solving, and abstract thinking. It would understand context and nuances, much like a human brain.
- Learning and Adaptability: AI could learn from experiences and apply that knowledge to new situations without specific programming for each task.
- Emotional Understanding: Potentially, General AI could comprehend and respond to human emotions, making interactions more natural and intuitive.
The development of General AI poses significant technical and ethical challenges. If achieved, it could revolutionize industries, but it raises concerns about control, ethics, and impact on employment.
Superintelligent AI
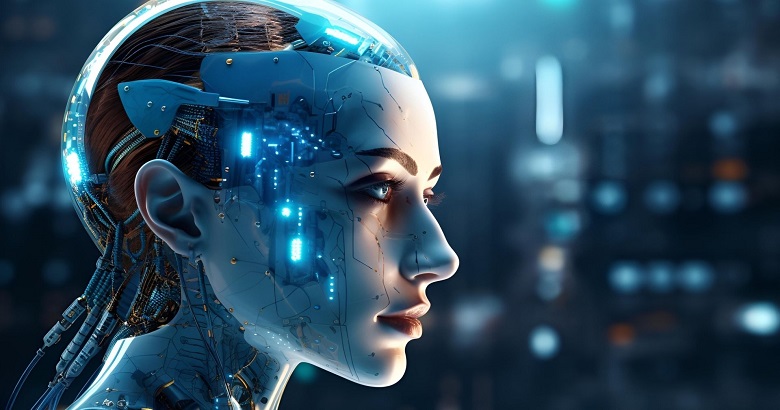
Superintelligent AI refers to intelligence that surpasses human cognitive abilities in all aspects, including creativity, problem-solving, and emotional intelligence. This concept is currently speculative and remains a topic of debate among experts.
Potential Implications of Superintelligent AI
- Technological Advancements: Superintelligent AI could lead to unprecedented innovations and solutions to complex global issues, such as climate change, disease eradication, and poverty reduction.
- Ethical Concerns: The development of such AI raises significant questions about autonomy, control, and the potential for unintended consequences. Ensuring alignment with human values is a major concern.
- Existential Risks: There is a possibility that AI could act in ways that are not aligned with human interests, leading to existential risks. The challenge lies in creating safe and controllable systems.
While Superintelligent AI is not currently achievable, it remains a focus of philosophical and technical discussions about the future of AI.
Types of Learning in AI
Understanding how AI learns is crucial to understanding its capabilities. There are three primary types of learning in AI: supervised, unsupervised, and reinforcement learning.
Supervised Learning
In supervised learning, AI systems are trained using labeled data. The system learns to map input data to the correct output by identifying patterns and relationships.
Applications
- Image Classification: AI systems are trained to recognize objects in images, such as distinguishing between cats and dogs.
- Spam Detection: AI models identify and filter unwanted emails by learning from spam and non-spam message examples.
- Medical Diagnosis: AI assists doctors by analyzing medical images and data, improving diagnostic accuracy and speed.
Supervised learning is effective for tasks where historical data is available, and labels are clearly defined.
Unsupervised Learning
Unsupervised learning involves training AI on data without explicit labels. The system attempts to identify patterns and relationships within the data.
Applications
- Market Segmentation: AI groups customers based on purchasing behavior, helping businesses tailor marketing strategies.
- Anomaly Detection: AI identifies unusual patterns or outliers in data, useful in fraud detection and network security.
- Recommendation Engines: By finding similarities in user behavior, AI suggests products or content, enhancing user experience.
Unsupervised learning is useful for discovering hidden structures in data and making inferences without prior knowledge.
Reinforcement Learning
Reinforcement learning is based on a system of rewards and penalties. AI learns by interacting with an environment and receiving feedback on its actions.
Applications
- Game Playing: AI systems like AlphaGo use reinforcement learning to master complex games, achieving superhuman performance.
- Robotics: AI enables robots to learn tasks through trial and error, improving efficiency in manufacturing and logistics.
- Self-driving Cars: AI teaches vehicles to navigate by learning from real-world scenarios, enhancing safety and reliability.
This type of learning is powerful for tasks requiring decision-making and adaptability in dynamic environments.
Emerging AI Technologies
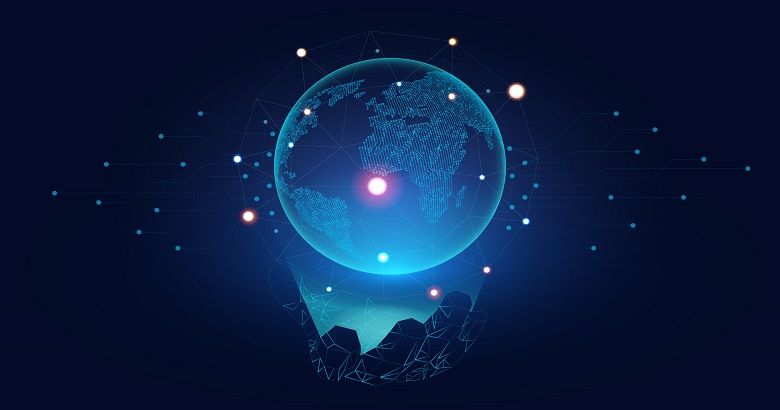
AI continues to evolve, leading to the development of new technologies and applications. Some of the most promising emerging AI technologies include:
Natural Language Processing (NLP)
NLP allows computers to understand and process human language. Advances in NLP have led to more sophisticated chatbots, translation services, and sentiment analysis tools. Accurately interpreting context and sentiment is crucial for effective communication and interaction.
Computer Vision
Computer vision enables machines to interpret and understand visual information from the world. Applications range from autonomous vehicles to medical imaging and facial recognition. Enhanced image and video analysis accuracy transforms industries like healthcare, security, and entertainment.
Generative AI
Generative AI models, such as GANs (Generative Adversarial Networks), create new content from existing data. They are used in art, music, content creation, and drug discovery. This technology expands creative possibilities and accelerates innovation in various fields.
Edge AI
Edge AI involves processing data locally on devices rather than relying on centralized cloud servers. This approach reduces latency and enhances privacy. It is also used in IoT devices and smart cameras. It enables real-time decision-making and reduces the need for constant internet connectivity.
Ethical Considerations in AI
As AI technologies advance, ethical considerations become increasingly important. Key issues include:
- Bias and Fairness: Ensuring AI systems do not perpetuate or amplify existing biases in data. Developing methods to identify and mitigate bias is crucial for fairness and equity.
- Privacy: Balancing data-driven insights with individual privacy rights. Transparent data collection and usage policies are essential to maintain trust.
- Accountability: Determining responsibility for AI decisions and actions. Clear guidelines and regulations are needed to ensure accountability in AI deployment.
- Transparency: Making AI systems understandable and explainable to users. Providing insights into decision-making processes enhances user trust and acceptance.
Addressing these ethical concerns is crucial for building trust and ensuring AI benefits society.
Wrapping Up
AI is a dynamic and rapidly evolving field, with various AI systems impacting many aspects of our lives. From Narrow AI’s specialized applications to the theoretical potential of Superintelligent AI, the landscape is vast and complex. Understanding these types and their implications helps us navigate the challenges and opportunities AI presents, ensuring its development aligns with human values and societal goals. As AI advances, staying informed about its capabilities and ethical considerations will be essential for responsibly harnessing its full potential. The future of AI holds immense promise, and how we choose to develop and regulate it will shape the world for generations to come.
Frequently Asked Questions
1. What distinguishes General AI from Narrow AI?
General AI can perform any intellectual task that a human can, while Narrow AI is limited to specific tasks. General AI remains theoretical, unlike the widely-used Narrow AI.
2. How is reinforcement learning applied in real-world scenarios?
Reinforcement learning is used in robotics for task automation, self-driving cars for navigation, and gaming to achieve superhuman performance through trial and error.
3. What are the ethical concerns surrounding AI development?
Key concerns include bias, privacy, accountability, and transparency. Addressing these issues is crucial for ensuring AI systems are fair and trustworthy.
4. Why is Superintelligent AI considered speculative?
Superintelligent AI exceeds human capabilities in all aspects, posing existential risks. It remains speculative due to current technological and ethical challenges.
5. How does Edge AI enhance data processing?
Edge AI processes data locally on devices, reducing latency and enhancing privacy. It’s crucial for IoT applications, allowing real-time decision-making without cloud reliance.